E-commerce has become an integral part of our lives. With the convenience of online
shopping, consumers are faced with an overwhelming number of options. Finding the
perfect product amidst this vast sea of choices can be a daunting task. However, thanks to
advancements in technology, product matching has emerged as a game-changer in the
e-commerce industry. So let us explore the concept of product matching and the benefits it
offers to both businesses and consumers.
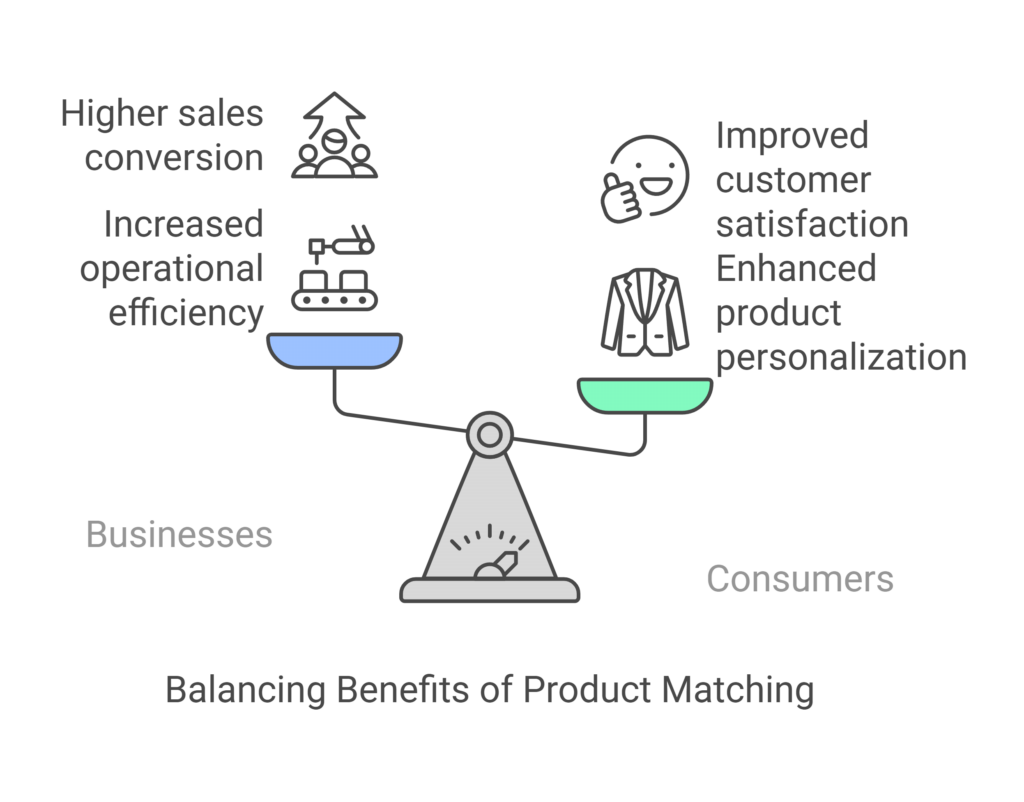
When it comes to product matching in e-commerce, several key features contribute to its
effectiveness and accuracy. These features are designed to enhance the shopping
experience for customers and provide businesses with valuable insights. Here are some key
features of product matching:
- Customizable Algorithmic Matching: Product matching relies on sophisticated algorithms that analyze various product attributes, such as titles, descriptions, specifications, and images. These algorithms use machine learning techniques to identify similarities and patterns, allowing for accurate product recommendations.
- Attribute-based Matching: Product matching algorithms consider multiple attributes of
products to find relevant matches. This includes factors such as size, color, brand, price
range, and specific features. By taking into account these attributes, the system can provide
precise matches based on user preferences. - Collaborative Filtering: Collaborative filtering is a technique that leverages user behavior
and preferences to generate product recommendations. By analyzing data from similar users,
the system identifies patterns and suggests items that align with a customer’s interests. This
approach is particularly useful for discovering new products or items that are popular among
like-minded individuals. - Natural Language Processing (NLP): NLP is used to understand and analyze product
descriptions, user reviews, and other textual information. By extracting meaningful insights
from text, product matching algorithms can identify relevant products and improve the
accuracy of recommendations. - Real-time Updates: E-commerce platforms continuously update their product databases
and inventory. Product matching systems are designed to handle these updates in real time,
ensuring that the recommendations are always up-to-date and reflective of the current
product availability. - Similarity Scoring: To determine the degree of similarity between products, matching
algorithms assign similarity scores. These scores indicate how closely a recommended
product matches the desired item. By considering these scores, businesses can prioritize and
present the most relevant and accurate recommendations to their customers. - Contextual Matching: Contextual matching takes into account the context in which a user
is browsing or searching for products. This includes factors such as the user’s location,
device, time of day, or browsing history. By considering these contextual cues, the system
can provide more tailored and localized recommendations. - Multi-Channel Matching: With the rise of omnichannel retail, product matching has
expanded to encompass various channels, including websites, mobile apps, and social media
platforms. This enables businesses to offer consistent and personalized recommendations
across different touchpoints, providing a seamless shopping experience for customers
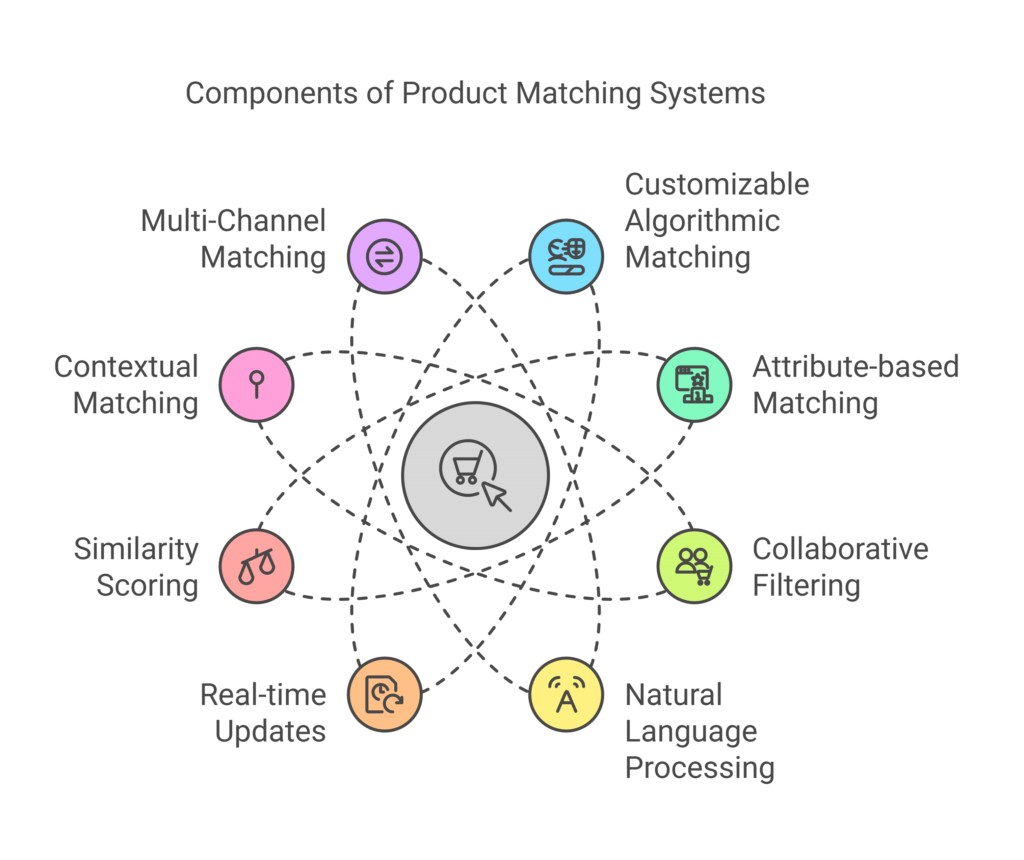
Understanding Product Matching:
Product matching refers to the process of identifying similar or related products based on
various attributes such as appearance, features, specifications, or user reviews. This advanced
technology employs algorithms and machine learning techniques to create accurate and
relevant matches, ensuring a seamless shopping experience for customers.
Product matching is a crucial process in the realm of e-commerce and retail, ensuring
accurate and efficient product identification. There are three primary approaches to product
matching: static, dynamic, and hybrid.
Static product matching involves creating a predefined set of rules and algorithms to match
products based on specific attributes such as product titles, descriptions, or unique
identifiers. This approach is effective for standardized and well-defined product catalogs but
may lack flexibility when dealing with variations or updates in product data.
Dynamic product matching, on the other hand, employs machine learning and artificial
intelligence techniques to automatically learn and adapt to changing data patterns. By
analyzing vast amounts of product data, dynamic matching algorithms can uncover complex
relationships and similarities between products, improving accuracy and scalability. This
approach is particularly beneficial for large-scale e-commerce platforms with extensive
product inventories.
Hybrid product matching combines the strengths of both static and dynamic approaches. It
leverages predefined rules to quickly match products based on known attributes while also
incorporating machine learning algorithms to handle more challenging cases. This hybrid
approach offers a balance between flexibility and efficiency, providing accurate matches
while adapting to evolving product data
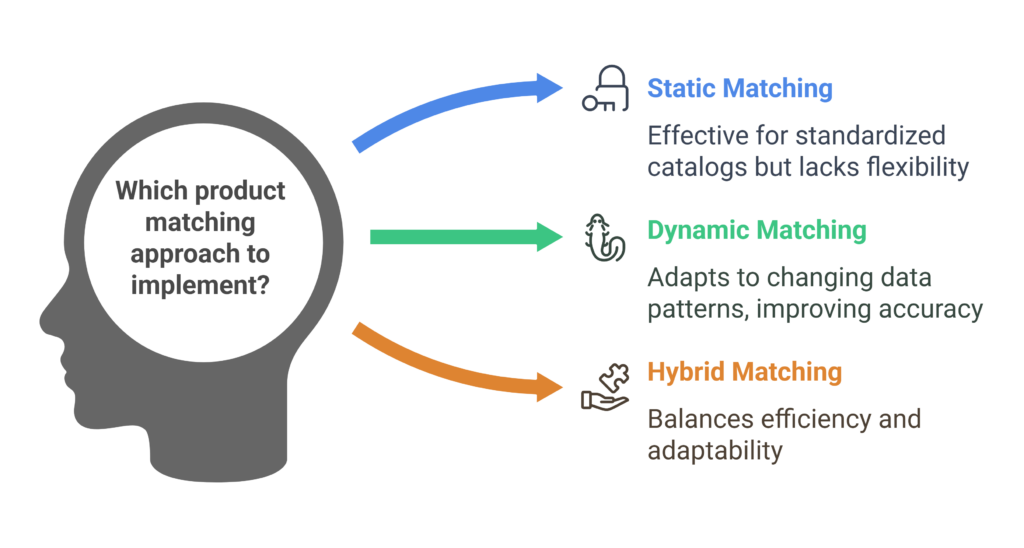
Benefits of Product Matching in E-commerce:
Product matching algorithms enable e-commerce platforms to offer personalized and
tailored recommendations to their customers. By analyzing user preferences and purchase
history, these systems can suggest products that align with individual tastes and
requirements. This personalized approach significantly enhances the overall shopping
experience, making it more enjoyable and efficient.
Improved Conversion Rates:
When customers are presented with accurate product matches, they are more likely to make
a purchase. By reducing the time and effort required to find the desired item, product
matching increases customer satisfaction and boosts conversion rates. This, in turn, translates
into higher sales and revenue for e-commerce businesses.
Enriched Customer Loyalty:
Effective product matching not only helps customers find what they are looking for but also
introduces them to new products they might not have discovered otherwise. By exceeding
customer expectations and providing relevant recommendations, e-commerce platforms can
build trust and loyalty among their user base. Satisfied customers are more likely to return for
future purchases, contributing to long-term business success.
Upselling and Cross-Selling Opportunities:
Product matching algorithms can identify complementary products or suggest alternatives to
the item being viewed. This opens up opportunities for upselling and cross-selling, where
customers are enticed to purchase related or higher-value items. By strategically
recommending additional products, e-commerce businesses can increase their average
order value and maximize revenue.
Inventory Optimization:
For e-commerce platforms managing a large inventory, product matching can play a vital
role in optimizing stock levels and minimizing wastage. By analyzing sales patterns and
identifying product associations, businesses can ensure that their inventory aligns with
customer preferences. This helps in reducing storage costs, minimizing the risk of stockouts,
and streamlining the supply chain.
Competitive Advantage:
In the fiercely competitive e-commerce landscape, standing out from the crowd is crucial. By implementing product matching technology, businesses can differentiate themselves by
offering a superior shopping experience. With accurate recommendations and personalized
suggestions, they can gain an edge over competitors and attract and retain more customers
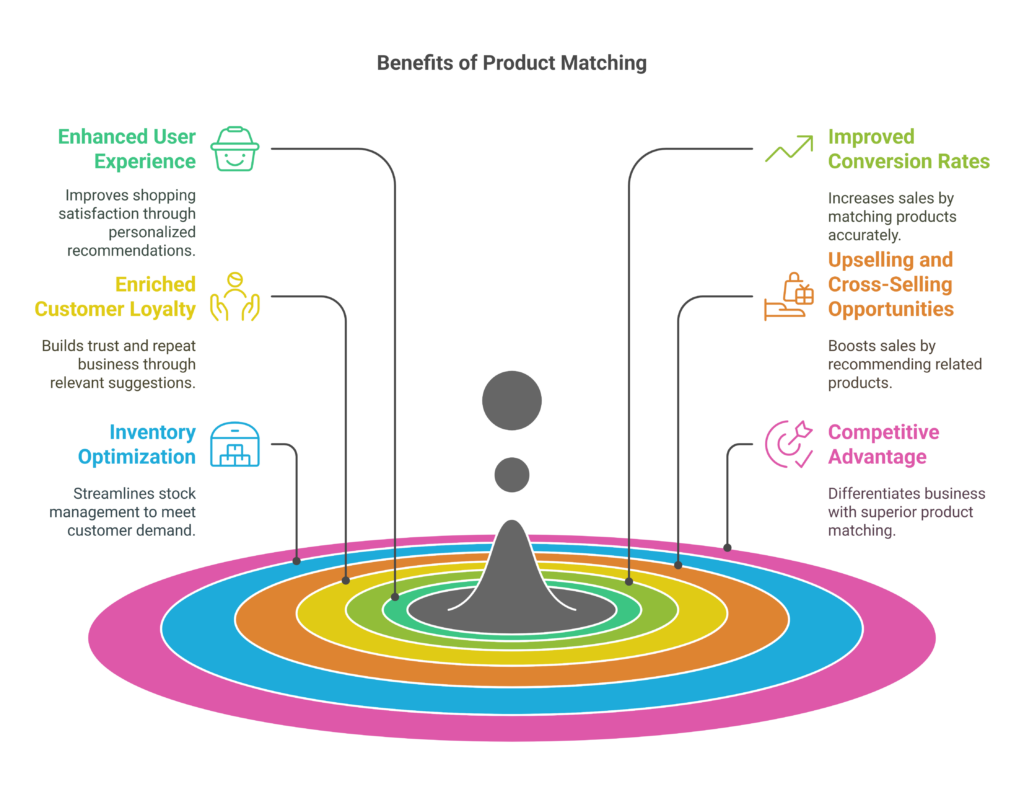
Conclusion:
Product matching has become a driving force behind the success of e-commerce businesses.
By harnessing the power of advanced algorithms and machine learning, product matching
enhances user experience, boosts conversion rates, increases customer loyalty, and creates
upselling opportunities. Moreover, it enables businesses to optimize their inventory and gain
a competitive advantage in the market. As e-commerce continues to evolve, product
matching will remain a vital tool in meeting customer expectations and driving growth in the
industry.